(This is a translation of my article in the Dutch printed magazine <!ELEMENT.)
The latest news on XQuery [1] was presented by me at the XML Holland conference [2] of 2010. All well, but what is the use of XQuery? And why use XQuery over other kinds of alternatives, XML-related or not? I’ll try to answer these questions in this article, and explain why the (relatively new) extensions to XQuery are so interesting.
What is the use of XQuery?
XQuery [3] stands for XML Query Language [4]. That already tells the essence. It is a language to select subsets and substructures from a large set of XML files. The result can be manipulated into something that is suitable to be used in, for example, a subsequent process, or to show in a web browser. XPath [5] is used a lot in XQuery.
All XML standards have their own scope. I’ll name a few. XSLT [6] is a language for transforming XML into some other format. XPointer [7] is an extension of XPath to address nodes more accurately within XML fragments or even subparts of nodes. XLink [8] is a standard to define relationships. XInclude [9] is a standard to compose multiple pieces of XML into one using for instance XLink relationships. And XProc [10] is a standard with which can be described how XML documents should be processed to get to a desired end result. It is expressed itself in XML, and describes the process step by step, also called XML Pipelines. Within XProc you use a.o. XQuery, XSLT, and XInclude languages (and thus indirectly XPath, XPointer and XLink as well) to express what needs to be done exactly within each step.
All these standards are tied together. They are related, and depend on each other. The overlap between some of the mentioned XML standards is summarized quite well in the next image that you can also find at W3Schools [11]:
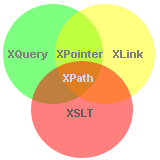
XQuery vs. XSLT
XQuery has originally a rather specific goal: extract XML fragments from a large(r) collection. This is very different from XSLT, which focusses on transforming XML documents into other XML documents, HTML documents or even documents of other formats.
You would think it should be pretty clear when and why you should use which standard. Yet we often hear the question whether it is best to use XSLT, or best to use XQuery. The point is that these two languages, more than the other ones, have a considerable overlap. There are many tasks you can do in XSLT, that you can also do in XQuery and vice versa. Although this question is in some ways unjustified and not always important, I'll discuss it in a little more detail below here.
If you can tackle something in multiple ways, and both ways do it with similar ease, there is no real reason for rejecting either of the two. Yet you will see that some people prefer XQuery. The syntax of XQuery is much more compact because it is not expressed in XML as XSLT. On the other hand, XSLT is based on a different principle, making doing for instance certain structural changes much easier. In this sense, it is mainly down to personal taste and the specific challenges of the task at hand, which of the two will be used by someone in particular for a given task.
However, XQuery is often used in combination with databases. That affects the balance. Firstly, XSLT fans aren’t always the same people who will be dealing with databases and vice versa. XSLT is more common in the area of document conversions. Secondly, databases entail additional challenges, often of an entirely different order of magnitude. XQuery has extensions that provide help in those areas. But there are no (official) XSLT extensions, and there is no real need for it either.
And that is why comparing XQuery and XSLT is so difficult, and therefore usually futile.
XQuery relatively unknown
The fact that XSLT exists much longer than XQuery, also affects the balance. In the beginning people had not much choice. Later on people got used to the quickly matured XSLT, while XQuery was still a working draft for quite some years. The idea for an "XML Query Language" arose along the emergence of XML, but it took long before it became a W3C Recommendation. XQuery is still relatively new, compared to XSLT and XPath.
One reason for this is that, after the launch of XPath in 1999, people soon became aware that such language could be largely based on XPath. That resulted in the first Working Draft of both XQuery 1.0 and XPath 2.0 in 2001. XSLT could and should of course also benefit. The XSLT 2.0 Working Draft was initiated at the same time. The Recommendations of these three were released more or less simultaneously. We are talking about 2007 by then, that is six years later!
So, XQuery is a Recommendation only since 2007, while XSLT and XPath are Recommendations since 1999, and were pretty popular from the start. XQuery is still catching up on XSLT and XPath. In addition, XML was booming business back then. Innovations in XML standards have slowed down, while new ideas like JSON [12] and NoSQL [13] are getting all the attention.
XQuery needs to catch up with XML databases as well. Various kinds of XML databases emerged after the advent of XML, but the idea of a generic Query Language didn’t appear until several years later. The fact that XQuery reached the Recommendation status only in the recent years, has slowed broad support in commercial database products the years before. A few large parties like IBM were involved in XQuery early on, other parties such as Oracle followed only years later. It was likewise with commercial XML databases: there were some early-adopters, but most of them preferred to wait to see which way the cat would jump.
Relation with databases
The fact that XQuery is used so often in combination with databases, is no coincidence. It is obvious to want to put large collections of XML in an (XML) database. Databases are designed for large-scale storage and efficient extraction. It fits the purpose of XQuery perfectly.
And that's no coincidence either. XQuery (indirectly) emerged out of database languages like SQL. The first ideas for storing XML in databases arose with the advent of XML. Initially people mainly (ab)used relational databases. However, languages like SQL are not equipped to handle XML. So, many extensions and variations arose automatically. By the time the XSLT and XPath Recommendations were a fact, people realized that there was a need for a generic query language as well. This resulted in the Quilt [14] language in 2000, which was renamed to XQuery after adoption by the W3C.
The following chart that I borrowed from sheets of a curriculum about XML and databases [15] (see ch. 10), shows briefly how various database languages merged into XQuery.
That is why it is no coincidence that XQuery and databases go so well together. XQuery is mainly designed and developed for use with databases. W3C has chosen explicitly not to limited it to only databases, making it more general purpose.
Relation with database functionality
Development around XQuery hasn’t stood still during all those years, though. There are quite a number of extensions to XQuery, which significantly increase the power of XQuery. Part of them find their origin in the application of XQuery to databases.
Ronald Bourret has a very informative website in which XML and databases [16] are elaborately discussed. He mentions some basic features that every database must support. Some of the more important are:
- Efficient storage and extraction
- (Full Text) Search
- Transactional updates
- Data integrity and triggers
- Parallel processing and access
- Security and crash recovery
- Version control of data
Storage is of course inherent to databases. A good database also provides facilities for concurrent access and updates, security, and crash recovery. Extraction is covered by XQuery 1.0, the search by the Full-Text standard, updates by the Update Facility standard. And there are extensions for data integrity and versioning as well, though yet unofficial. More on that in the following part.
Extensions on XQuery
XQuery 1.0 relies on XPath 2.0. It is in fact an extension to it. Even XPath, how powerful itself, has certain limitations. It is a language for addressing substructures. It is not really designed for searching. XQuery itself doesn’t provide the right functionality for searching either. It is designed for retrieval and processing. Therefore, an extension to these languages was developed: the "XQuery and XPath Full Text 1.0 [17]" standard, which became a W3C Recommendation [18] in March this year.
XQuery is meant for extraction and processing, not for applying changes. Another extension which became a W3C Recommendation in March this year is the "XQuery Update Facility 1.0 [19]" standard. This is an extension that does allow applying (permanent) changes to XML structures.
Regarding data integrity an (unofficial) proposal [20] was presented at the XML Prague 2010 conference [21]. This extension allows embedding declarations of data collections, indexes and data constraints within your XQuery code. Instead of having to mess around with database configurations, these declarations become part of the application code itself. This makes maintenance much easier. All relevant details gathered in one spot, and within control of the developer him/herself. They would not even need to know much about the database that is actually being used.
Versioning is commonly used for Content Management, but is also used for other purposes such as traceability. Another (unofficial) proposal [22] presented at XML Prague 2010 covers versioning. It is a bit technical, and goes quite deep, but it provides some interesting features. According to the Update Facility standard, all mutations are collected in a so-called ‘Pending Update List’. At the end of an updating script the result of all mutations in that script are committed (stored). This extension describes the idea to preserve all these ‘commit’ moments. To do this effectively, the proposal mentions something called ‘Pending Update List compositions’. These commit moments provide a full history of the XML. Two new XPath ‘axes’ are added, allowing navigation through the full history as integral part of XPath navigation.
Storing all this versioning data requires a lot of disk space, but it is such cheap these days that costs are no longer a problem.
Beyond Scope
But XQuery goes even further. There are currently two extensions that go way beyond database functionality.
The successor of XQuery 1.0 is being developed as we speak: XQuery 1.1, or actually XQuery 3.0 [23], which currently has the W3C Working Draft status. This successor adds a number of features that significantly enhance the expressiveness, such as: try / catch constructs, output statements, group by within a for loop. It also allows calling functions dynamically. In other words: functions as a data type. This takes XQuery to a whole new level.
And as if that were not enough, a standard called "XQuery Scripting Extension 1.0 [24]" is being developed as well. This extension adds several new features that almost make it a (procedural) programming language, for instance: a while loop, redefinition of ,variables and an exit statement. It also builds on top of the XQuery Update Facility standard and allows cumulative (sequential) updates.
All of this makes XQuery very suitable as a ‘scripting’ language, allowing it to compete with languages such as JSP, ASP and PHP. In fact when speaking of web applications it can compete with languages like Java and .Net equally well. It is not for nothing that W3C states:

Note: that is an observation, not an opinion!
Programming Language
XQuery 3.0 and the Scripting Extension lift XQuery to a higher level. They give the appearance of a real programming language. It is not a surprise that W3C states that database-specific programming languages are being replaced by XQuery more and more. XQuery is ideally suited as a language for database access, but thanks to these latest enhancements it goes further. XQuery is the glue that can bring all application layers together. It is also powerful enough to support well known Design Patterns [26] without much trouble. Not only the well-known Model-View-Controller [27] pattern, but also other useful patterns, such as Observer, Strategy and others [28].
It is easiest to refer to the application that me and two of my (former) colleagues have made for a programming contest [29] to show the real power of XQuery. The goal was simple: create an application that appeals to XQuery and was well put together. The result was Socialito [30]: a ‘Social Media Dashboard’, in which tweets and other information from your Twitter account is displayed in a highly organized, and customizable manner. The user interface uses HTML and JavaScript (JQuery [31]), but apart from that it uses XQuery exclusively. The data is stored using the XML structure of Twitter itself.
In short, XQuery is not just for "Querying XML" no longer. In XQuery, you can develop application logic and application layers all together. That makes it the core of your entire application. This goes way further than any other XML standard.
Learn more?
Anyone interested in learning more, and keen to see practical applications of XQuery, is kindly invited to sign up for the XML Amsterdam conference of Wednesday 26th of October at the Regardz Planetarium in Amsterdam. Several Open Standards will be discussed, and there will be multiple presentations on XQuery.
- latest news on XQuery: http://xmlholland.nl/sites/default/files/Geert Josten-XMLHolland2010.pdf
- XML Holland conference: http://www.xmlholland.nl/jaarcongres
- XQuery: http://www.w3.org/TR/xquery/
- XML Query Language: http://www.w3.org/XML/Query/
- XPath: http://www.w3.org/TR/xpath20/
- XSLT: http://www.w3.org/TR/xslt20/
- XPointer: http://www.w3.org/TR/xptr-framework/
- XLink: http://www.w3.org/TR/xlink11/
- XInclude: http://www.w3.org/TR/xinclude/
- XProc: http://www.w3.org/TR/xproc/
- W3Schools: https://www.w3schools.com/xml/xpath_intro.asp
- JSON: http://en.wikipedia.org/wiki/JSON
- NoSQL: http://en.wikipedia.org/wiki/NoSQL
- Quilt: http://xml.coverpages.org/quilt_euro.html
- XML and databases: http://www.inf.uni-konstanz.de/dbis/teaching/ws0708/xml/
- XML and databases: http://www.rpbourret.com/xml/XMLAndDatabases.htm
- XQuery and XPath Full Text 1.0: http://www.w3.org/TR/xpath-full-text-10/
- W3C Recommendation: http://www.w3.org/TR/
- XQuery Update Facility 1.0: http://www.w3.org/TR/xquery-update-10/
- proposal: http://www.xmlprague.cz/2010/presentations/Matthias Brantner Extending_XQuery_with_Collections_Indexes_and_Integrity_Constraints.pdf
- XML Prague 2010 conference: http://www.xmlprague.cz/2010/index.html
- proposal: http://www.xmlprague.cz/2010/sessions.html
- XQuery 3.0: http://www.w3.org/TR/xquery-30/
- XQuery Scripting Extension 1.0: http://www.w3.org/TR/xquery-sx-10/
- http://www.w3.org/XML/Query/: http://www.w3.org/XML/Query/
- Design Patterns: http://en.wikipedia.org/wiki/Design_pattern_(computer_science)
- Model-View-Controller: http://code.google.com/p/xqmvc/
- Observer, Strategy and others: http://patterns.28msec.com/
- Programming contest: http://www.28msec.com/contest/results
- Socialito: http://socialito.my28msec.com/
- JQuery: http://jquery.com/
- Personal blog: http://grtjn.blogspot.com/
- Company blog: http://www.daidalos.nl/blogs/blog/author/Geert/